A few years ago, we wrote a blogpost about the Commander framework, where we explained how the setpoint structure worked, which drives the controller of the Crazyflie, which is an essential part of the stabilization module. Basically, without these, there would not be any autonomy on the Crazyflie, let alone manual flight.
In the blogpost, we already shed some light on where different setpoints can come from in the commander framework, either from the Crazyflie python library (externally with the Crazyradio), the high level commander (onboard) or the App layer (onboard).
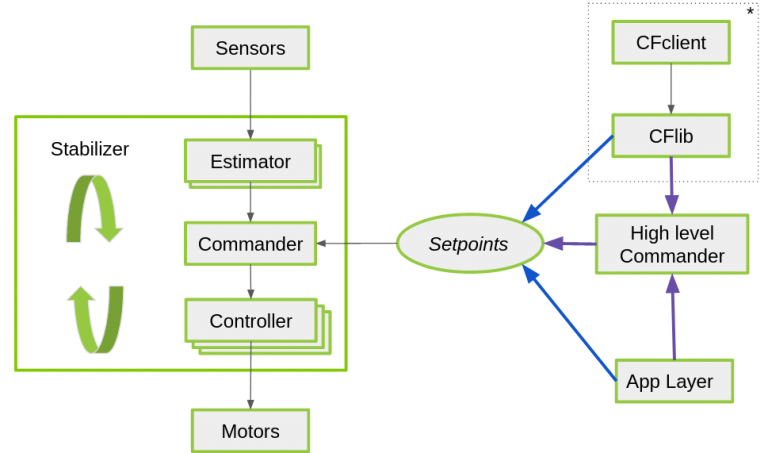
However, we notice that there is sometimes confusion regarding these different functionalities and what exactly sends which setpoints and how. These details might not be crucial when using just one Crazyflie, but become more significant when managing multiple drones. Understanding how often your computer needs to send setpoints or not becomes crucial in such scenarios. Therefore, this blog post aims to provide a clearer explanation of this aspect.
Sending set-points directly from the CFlib
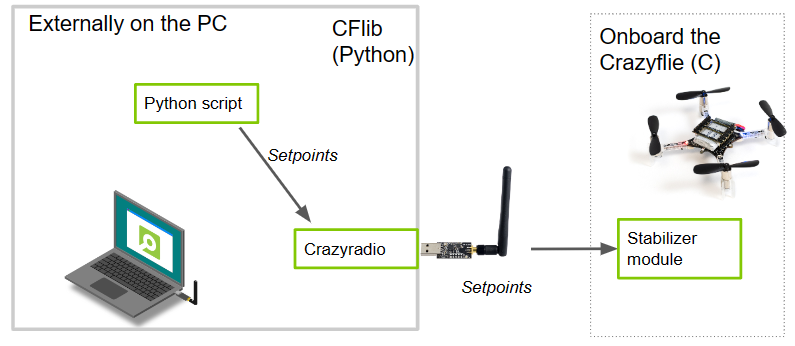
Let’s start at the lower level from the computer. It is possible to send various types of setpoints directly from a Python script using the Crazyflie Python library (cflib for short). This capability extends to tasks such as manual control:
send_setpoint(roll, pitch, yawrate, thrust)
or for hover control (velocity control):
send_hover_setpoint(vx, vy, yawrate, zdistance)
You can check the automatic generated API documentation for more setpoint sending options.
If you use these functions in a script, the principle is quite basic: the Crazyradio sends exactly 1 packet with this setpoint over the air to the Crazyflie, and it will act upon that. There are no secret threads opening in the background, and nothing magical happens on the Crazyflie either. However, the challenge here is that if your script doesn’t send an updated setpoint within a certain amount of time (default of 2 seconds), a timeout will occur, and the Crazyflie will drop out of the sky. Therefore, you need to send a setpoint at regular intervals, like in a for loop, to keep the Crazyflie flying. This is something you need to take care of in the script.
Example scripts in the CFlib that are sending setpoints directly:
- autonomy/autonomousSequence.py: Sending send_position_setpoint() in a loop:
- aideck/fpv.py: Sending send_hover_setpoint() based on keyboard commands.
- autonomy/full_state_setpoint_demo.py: Sending send_full_state_setpoint()
- swarm/swarmSequence.py: Sending send_position_setpoint() for a swarm
- swarm/swarmSequenceCircle.py: Sending send_hover_setpoint() for a swarm
Setpoint handling through Motion Commander Class
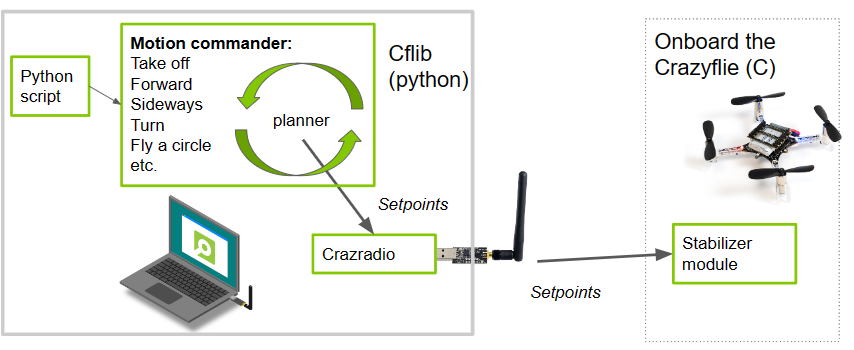
Another way to handle the regular sending of setpoints automatically in the CFLib is through the Motion Commander class. By initializing a Motion Commander object (usually using a context manager), a thread is started with takeoff that will continuously send (velocity) setpoints at a fixed rate. These setpoints can then be updated by the following functions, for instance, moving forward with blocking:
forward(distance)
or a giving body fixed velocity setpoint updates (that returns immediately):
start_linear_motion(vx, vy, vz, rate_yaw)
You can check the Motion Commander’s API-generated documentation for more functions that can be utilized. As there is a background thread consistently sending setpoints to the Crazyflie, no timeout will occur, and you only need to use one of these functions for the ‘behavior update’. This thread will be closed as soon as the Crazyflie lands again.
Here are example scripts in the CFlib that use the motion commander class:
- autonomy/motion_commander_demo.py: Showing off the different functions available for the motion commander.
- step-by-step/sbs_motion_commander.py: Showing off the different functions available for the motion commander.
- multiranger/multiranger_push.py: Using start_linear_motion(vx, vy, vz)
- multiranger/multirnager_wall_following.py: Using start_linear_motion(vx, vy, vz)
Setpoint handling through the high level commander
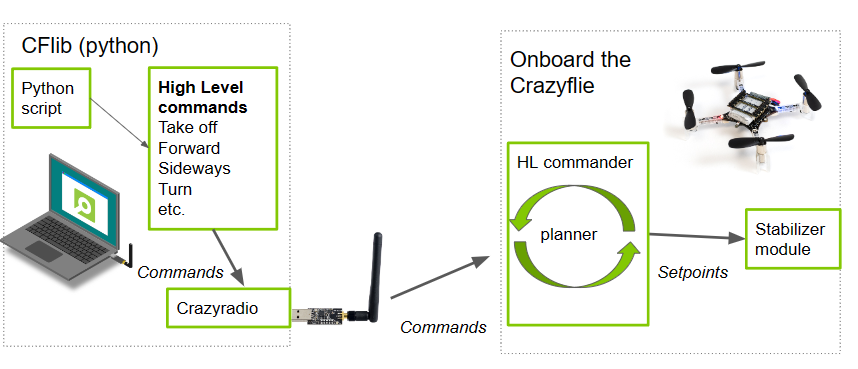
Prior to this, all logical and setpoint handling occurred on the PC side. Whether sending setpoints directly or using the Motion Commander class, there was a continuous stream of setpoint packets sent through the air for every movement the Crazyflie made. However, what if the Crazyflie misses one of these packets? Or how does this stream handle communication with many Crazyflies, especially in swarms where bandwidth becomes a critical factor?
This challenge led the developers at the Crazyswarm project (now Crazyswarm2) to implement more planning autonomy directly on the Crazyflie itself, in the form of the high-level commander. With the High-Level Commander, you can simply send one higher-level command to the Crazyflie, and the intermediate substeps (setpoints) are generated on the Crazyflie itself. This can be achieved with a regular takeoff:
take_off(height)
or go to a certain position in space:
go_to(x, y)
This can be accomplished using either the PositionHLCommander, which can be used as a context manager similar to the Motion Commander (without the Python threading), or by directly employing the functions of the High-Level Commander. You can refer to the automated API documentation for the available functions of the PositionHLCommander class or the High-Level Commander class.
Here are examples in the CFlib using either of these classes:
- autonomy/autonomous_sequence_high_level.py: Using the high level commander class directly
- autonomy/position_commander_demo.py: Using the PositionHLCommander class as context manager.
- swarm/hl-commander-swarm.py: Using the high level commander functions directly with a swarm
- swarm/synchronizedSequence.py: Using the high level commander functions directly with a swarm
Notes on location of autonomy and discrepancies
Considering the various options available in the Crazyflie Python library, it’s essential to realize that these setpoint-setting choices, whether direct or through the High-Level Commander, can also be configured through the app layer onboard the Crazyflie itself. You can find examples of these app layer configurations in the Crazyflie firmware repository.
It’s important to note some discrepancies regarding the Motion Commander class, which was designed with the Flow Deck (relative positioning) in mind. Consequently, it lacks a ‘go to this position’ equivalent. For such tasks, you may need to use the lower-level send_position_setpoint() function of the regular Commander class (see this ticket.) The same applies to the High-Level Commander, which was primarily designed for absolute positioning systems and lacks a ‘go forward with x m/s‘ equivalent. Currently, there isn’t a possibility to achieve these functionalities at a lower level from the Crazyflie Python library as this functionality needs to be implemented in the Crazyflie firmware first (see this ticket). It would be beneficial to align these functionalities on both the CFlib and High-Level Commander sides at some point in the future.
Hope this helps a bit to explain the commander frame work in more detail and where the real autonomy lies of the Crazyflie when you use different commander classes. If you have any questions on what the Crazyflie can do with these, we advise you to ask your questions on discussions.bitcraze.io and we will try to point you in the right direction and give examples!